The Role of Machine Learning in Drug Discovery
By Caleigh Findley
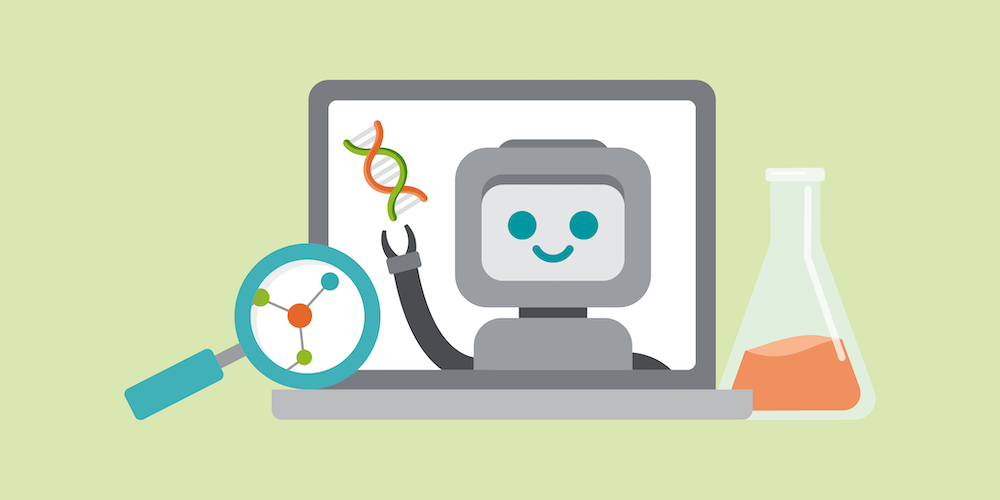
Investigating one preclinical drug candidate and disease at a time is a thing of the past. This traditional approach drags out the discovery process, spending too much time in development and delaying progress into clinical trials. It doesn’t have to be this way.
Drug hunting is transforming thanks to machine learning and sophisticated drug discovery platforms. Researchers now have the power to identify many drug candidates at once, optimize their lead compounds, and streamline the entire drug development process.
Read on to learn about how to leverage data platforms to maximize efficiency in drug discovery:
The Problem: Traditional Drug Hunting is Too Slow and Costly
Historically, bringing a new drug to market meant 10-15 years of research and costs of $2 billion or more. All that labor and investment toward a lead compound that may not make it out of clinical trials, as roughly 90% of compounds fail. This approach left too many diseases without effective therapies, creating a significant unmet need for innovation. In a time where machine learning has optimized many aspects of work for scientists, drug discovery is no exception.
The Solution: Optimize with Machine Learning Algorithms
Machine learning algorithms have emerged as indispensable tools in predicting the pharmacokinetics, toxicity, and efficacy of compounds, catapulting drug discovery into a new era of efficiency and accuracy. By leveraging vast datasets and complex algorithms, researchers can forecast experimental outcomes with unprecedented precision, significantly reducing the time and resources required for preclinical development and clinical trials.
Precision in Pharmacokinetics
One of the critical challenges in drug development is understanding how a compound interacts with the body, particularly its absorption, distribution, metabolism, and excretion (ADME) properties. Machine learning algorithms analyze diverse biological data to predict pharmacokinetic parameters, enabling researchers to prioritize compounds with optimal absorption rates, bioavailability, and metabolic stability. This targeted approach not only expedites the selection of lead compounds but also minimizes the risk of unexpected adverse effects during later stages of development.
Mitigating Toxicity Risks
The identification of potential toxicities is a critical aspect of drug safety assessment. Traditional methods rely heavily on animal testing and empirical observations, which are time-consuming and ethically challenging. Machine learning algorithms, however, can discern intricate patterns in chemical structures and biological responses to predict toxicity profiles with remarkable accuracy. By flagging compounds with high likelihoods of toxicity early in the discovery process, researchers can prioritize safer alternatives and avert costly setbacks in later stages of development.
Maximizing Efficacy
Achieving therapeutic efficacy is the ultimate goal of drug discovery, yet it remains elusive for many drug candidates because of complex interactions within biological systems. Machine learning algorithms analyze multi-dimensional data, including molecular structures, target proteins, and disease pathways, to predict the efficacy of compounds against specific therapeutic targets. This predictive modeling accelerates the identification of promising drug candidates and facilitates the design of targeted therapies tailored to individual patient profiles, ushering in a new era of personalized medicine.
Empowering Faster Discovery
Integrating machine learning into drug discovery processes offers unparalleled scale and efficiency, revolutionizing the pace at which new therapeutics are identified and developed. By harnessing the power of artificial intelligence to sift through vast datasets, researchers can uncover hidden correlations, identify novel drug targets, and expedite the optimization of lead compounds. Moreover, the iterative nature of machine learning enables continuous refinement of predictive models, enhancing their accuracy and reliability over time.
Implementation Challenges
While large data platforms backed by machine learning can be effective tools for drug hunting, they have limitations. Many considerations are involved when building such a platform, such as the amount (and quality!) of data used during the initial training period. Utilizing an inadequate dataset may impair the accuracy of your data platform, which will cause additional issues if implemented in experiments.
Federal regulators are also working to keep up with the transition toward machine learning for drug discovery. The Food and Drug Administration is currently consulting with experts and hosting discussions to inform regulatory guidelines for drug-hunting data platforms in pharmaceutical development.
Summary
Machine learning has emerged as a catalyst for transformation in drug discovery. By harnessing the predictive power of advanced computational algorithms, researchers can navigate the intricate landscape of molecular interactions with unprecedented precision and efficiency. From predicting pharmacokinetics and mitigating toxicity risks to maximizing therapeutic efficacy, the applications of machine learning in drug discovery are vast and far-reaching. This synergy between human ingenuity and artificial intelligence holds the promise of unlocking new frontiers in medicine, bringing hope to patients and clinicians alike.